Breaking New Ground in Dermatology: How Multi-Task AI is Transforming Skin Lesion Diagnosis
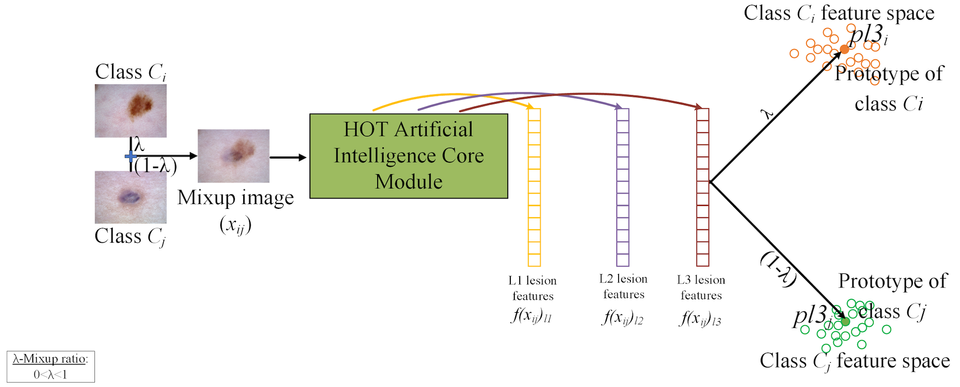
In the rapidly evolving world of medical AI, one area gaining momentum is dermatology, where early detection of skin lesions can make the difference between life and death. However, traditional AI models often fall short when faced with the complex realities of real-world diagnosis. A groundbreaking approach developed by researchers at Monash University seeks to change that narrative [1]. Enter the HOT AI model — a multi-task framework designed to overcome critical limitations in skin lesion diagnostics.
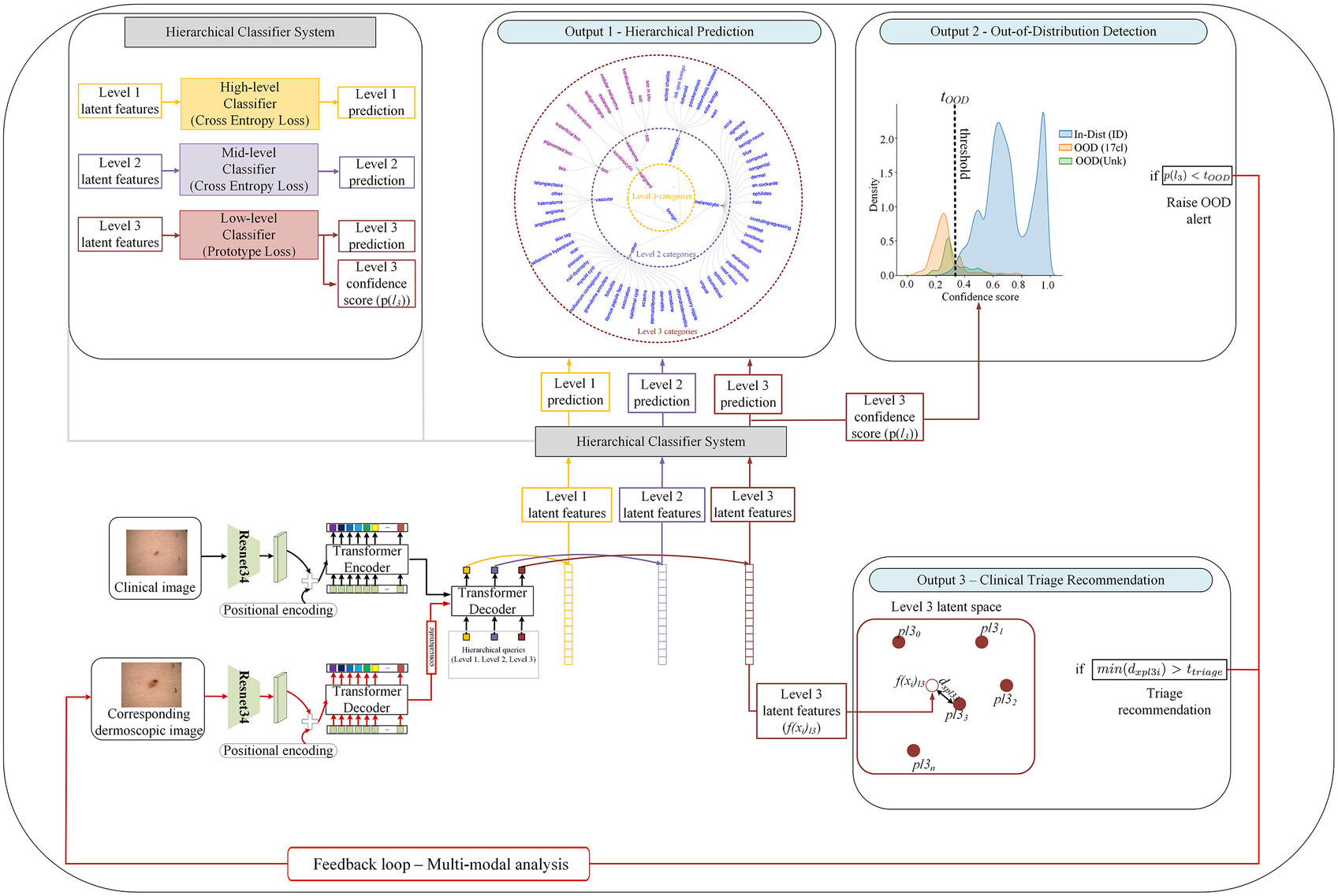
Why Traditional Models Fall Short
Conventional AI systems used in dermatology are often trained in ideal, controlled conditions using dermoscopic images and binary classification outputs (e.g., malignant vs. benign). But this doesn't translate well into the real world, where patients present with a spectrum of conditions, and clinical images vary significantly in quality and context.
Three major shortcomings are prevalent in standard models:
- Over-reliance on dermoscopy: Many models assume dermoscopic imaging is always available, which is not the case in many clinical settings.
- Limited output: Binary classification oversimplifies the diagnostic process and provides little clinical utility.
- Struggles with rare conditions: Traditional models often perform poorly with underrepresented or rare lesion categories.
The HOT AI Framework: A Multi-Task Powerhouse
To address these challenges, the researchers introduced the HOT AI model—a hierarchical, out-of-distribution-aware, and clinically adaptive framework that mimics how clinicians think and operate.
The model integrates three critical functions:
- Hierarchical diagnosis across three levels (benign/malignant, category type, and specific lesion class)
- Out-of-distribution (OOD) detection, which flags unfamiliar or rare lesion types
- Dermoscopy recommendation, suggesting when higher-resolution imaging is likely to improve accuracy
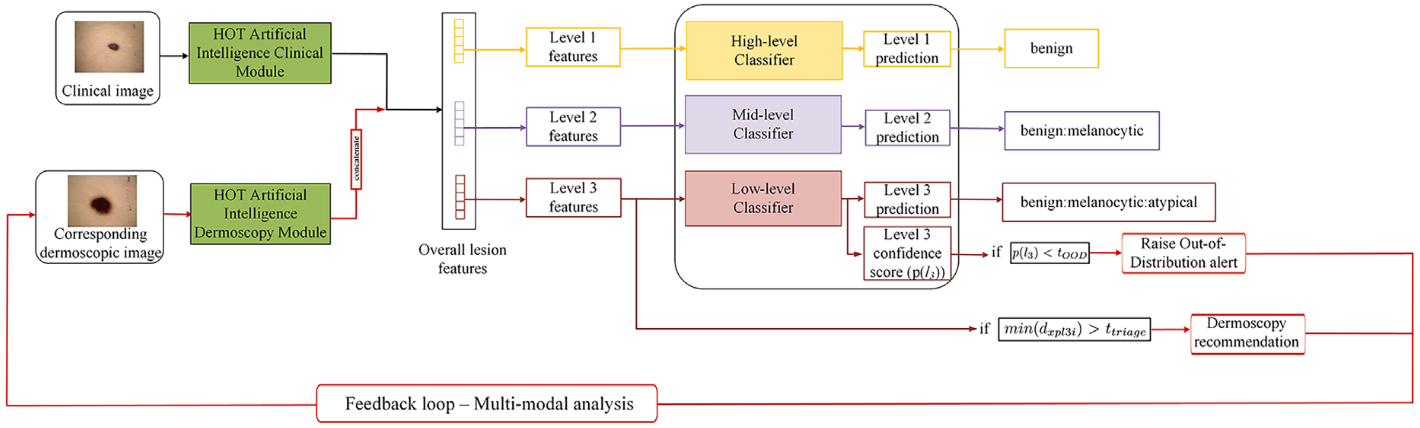
Training on a Massive, Real-World Dataset
The model was trained using the Molemap dataset [2], comprising over 208,000 images from nearly 79,000 patients, collected from 141 clinics across Australia and New Zealand. Importantly, it includes both clinical and dermoscopic images, offering a balanced view of real-world scenarios.
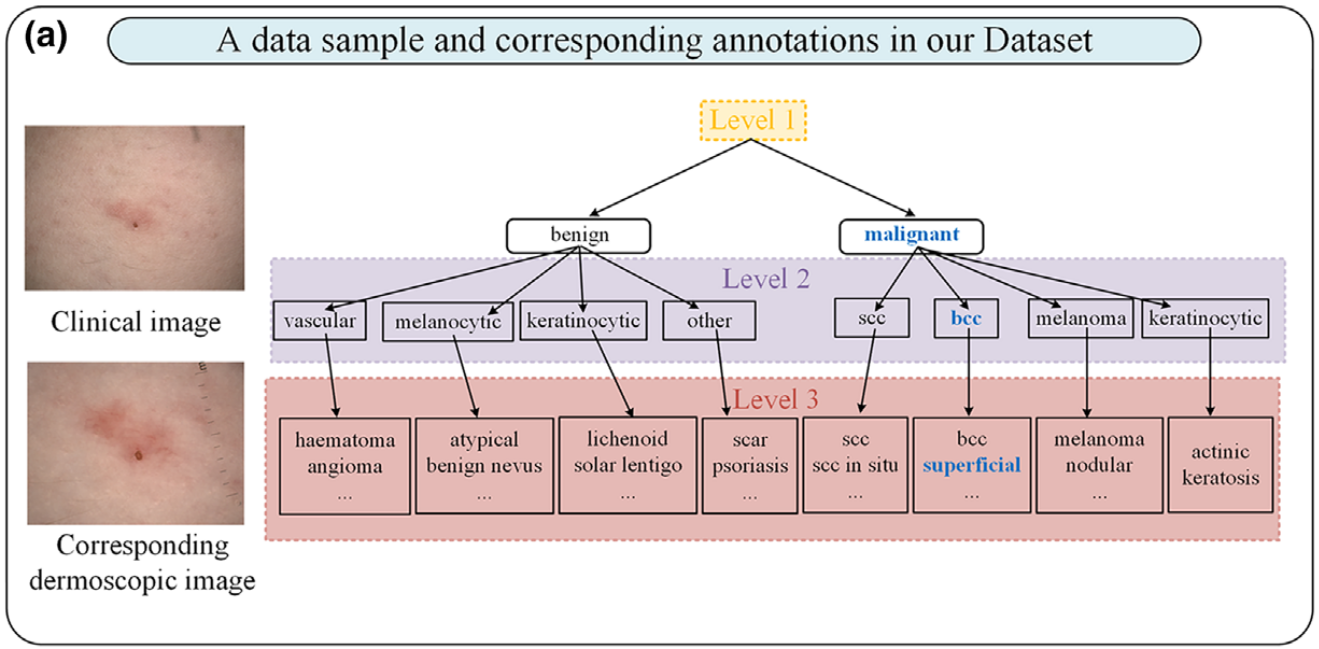
To simulate real-world deployment, the dataset was carefully split across three configurations:
- Random split: Standard method for model training
- Patient split: Ensures that no patient appears in both training and testing sets
- Clinic split: Ensures geographic and procedural diversity in data origin
The Science Behind HOT AI
The model architecture includes two main components:
1. Hierarchical Module
This module uses a combination of a ResNet34 CNN and DEtection TRansformer (DETR) [5] to deliver predictions at three diagnostic levels:
- Level 1: Benign vs. malignant
- Level 2: Eight mid-level diagnostic categories (e.g., melanocytic, keratinocytic)
- Level 3: 44 fine-grained lesion categories
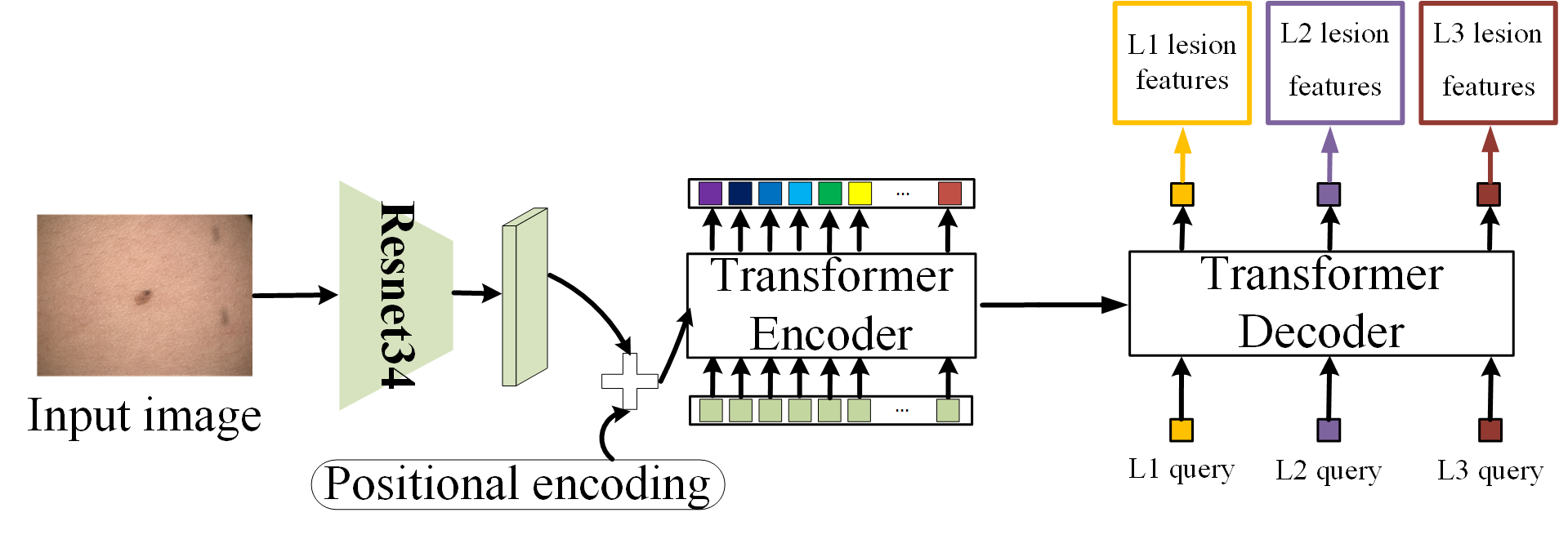
This approach mimics the diagnostic pathway a human dermatologist might follow, starting broad and narrowing down.
2. Mixup and Prototype Learning (MPL) Module
To boost generalization and interpretability, the MPL module introduces two novel strategies:
- Mixup [3]: Generates new training samples by combining image pairs, improving robustness
- Prototype Learning [4]: Represents each class by a prototype in feature space, ensuring new examples are close to their class centers and far from others
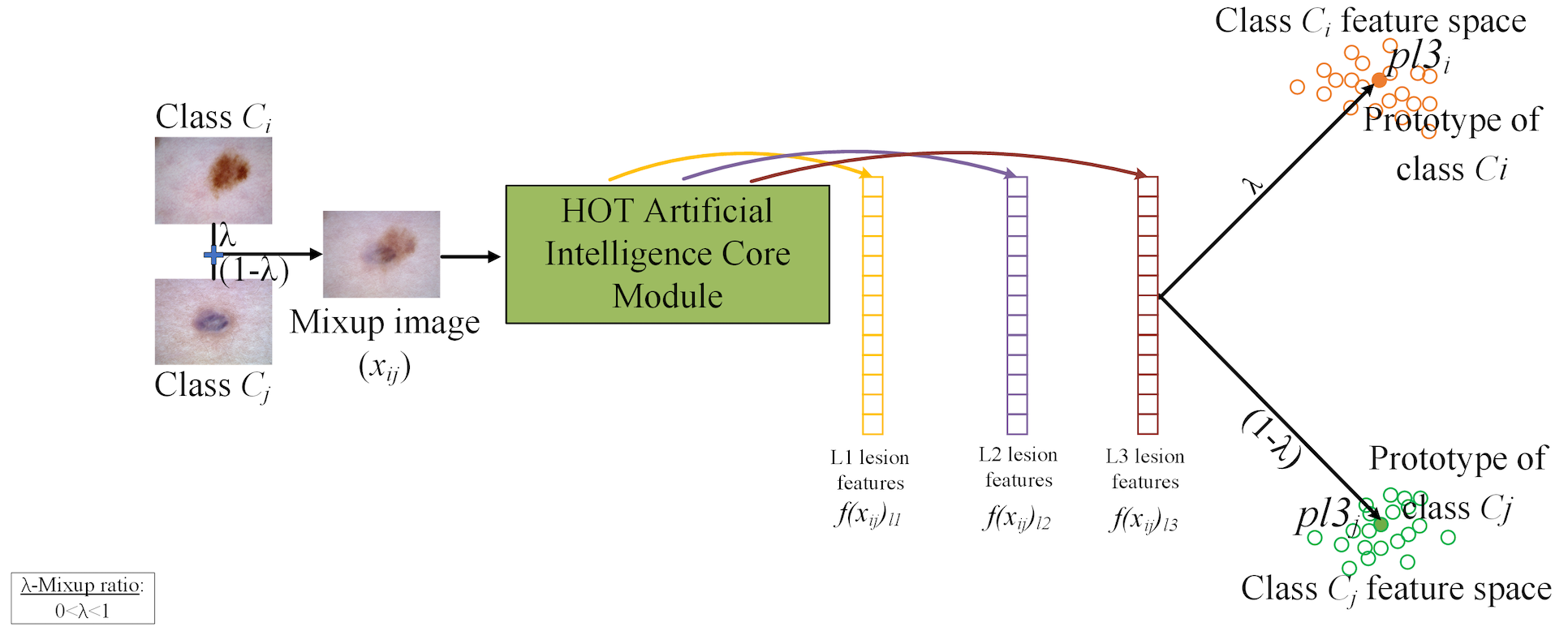
This module also handles OOD detection and clinical triage recommendations based on how far a new input's features deviate from known prototypes.
Smarter Predictions, Safer Diagnoses
What makes HOT AI a game-changer is its ability to avoid misdiagnoses through multi-level prediction. If the model is unsure at the fine-grained level, it can still provide reliable higher-level categories to guide decision-making. This is particularly useful in ambiguous cases.
Additionally, the triage recommendation system has proven effective. It identifies cases where dermoscopic imaging would significantly improve accuracy, increasing true-positive predictions in:
- 72.02% of cases (random split)
- 87.4% (patient split)
- 70.03% (clinic split)
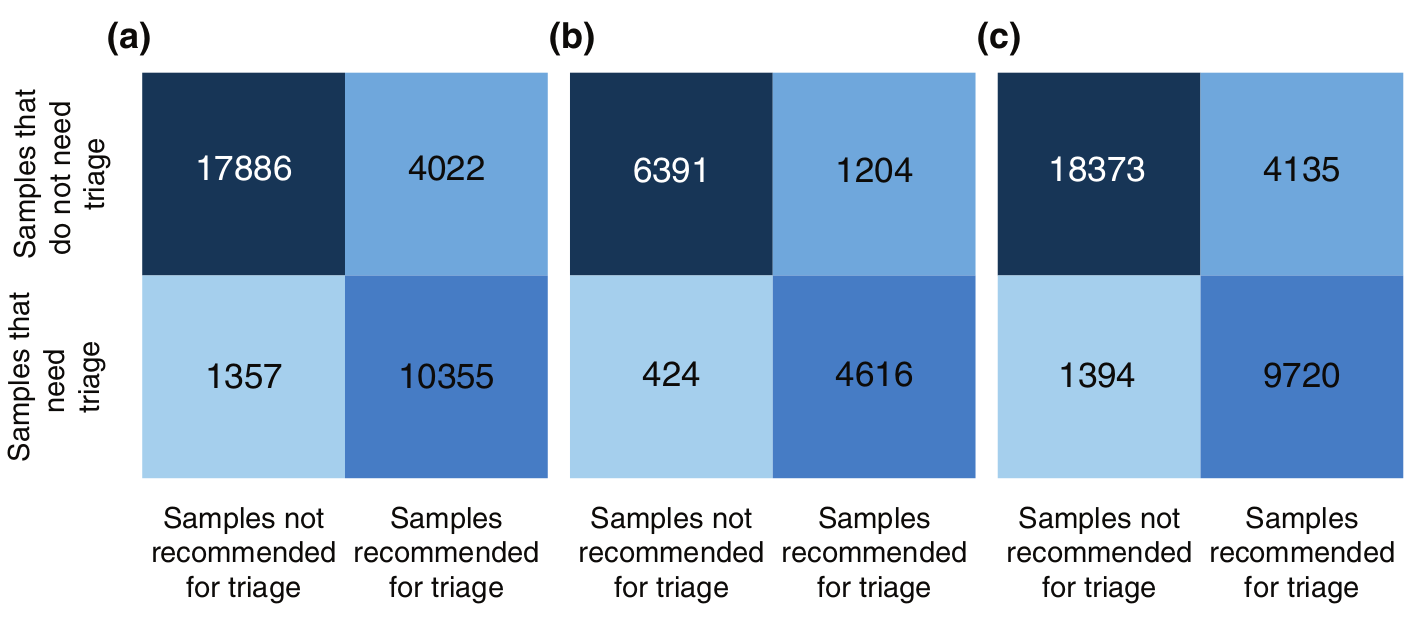
Conversely, it avoids unnecessary imaging in over 92% of non-critical cases.
Outshining the State-of-the-Art
In head-to-head comparisons with leading dermatology AI systems, HOT AI consistently came out on top. Notably, it delivered:
- Higher sensitivity and specificity for Level 3 classifications
- Superior OOD detection accuracy, outperforming all benchmarked models across clinical, dermoscopic, and mixed modalities
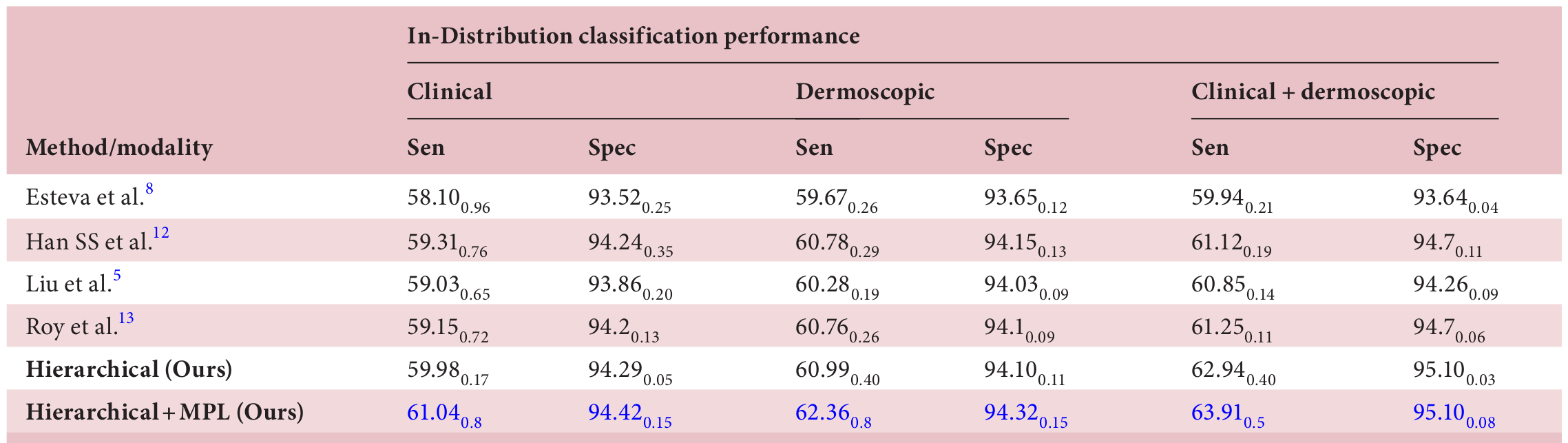
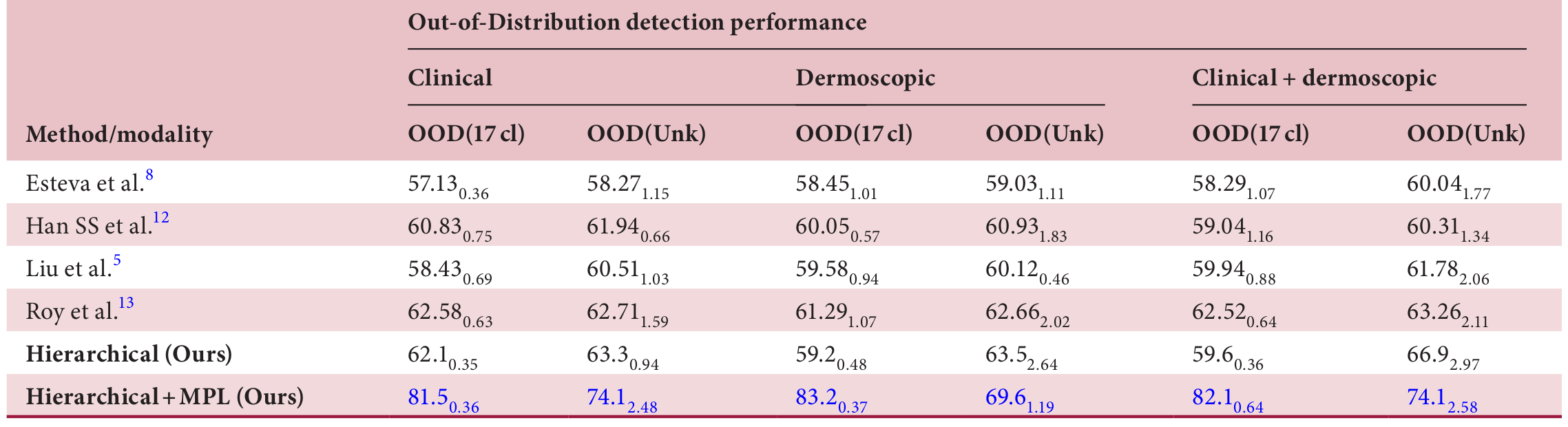
Final Thoughts
The HOT AI model represents a significant advancement in AI-driven dermatology. By embracing complexity through hierarchical learning, proactively managing rare conditions with OOD detection, and offering intelligent clinical guidance, it sets a new standard for what diagnostic AI can achieve.
Yet, the journey isn’t over. As emphasized in the conclusion, prospective clinical trials are needed to validate the model’s real-world effectiveness and its impact on user trust and decision-making.
Still, this multi-task model offers a glimpse into the future of dermatological diagnostics—one where AI doesn’t just assist, but empowers clinicians to deliver smarter, safer care.
References
- Mehta, D., Primiero, C., Betz-Stablein, B., Nguyen, T. D., Gal, Y., Bowling, A., et al. (2025). Multi-task AI models in dermatology: Overcoming critical clinical translation challenges for enhanced skin lesion diagnosis. Journal of the European Academy of Dermatology & Venereology (JEADV). https://onlinelibrary.wiley.com/doi/pdf/10.1111/jdv.20551
- Molemap dataset. Clinical and dermoscopic images collected from community clinics in Australia and New Zealand (2005–2020). https://www.molemap.net/
- Zhang, H., Cisse, M., Dauphin, Y. N., & Lopez-Paz, D. (2018). mixup: Beyond Empirical Risk Minimization. ICLR.
https://arxiv.org/abs/1710.09412 - Snell, J., Swersky, K., & Zemel, R. S. (2017). Prototypical Networks for Few-shot Learning. NeurIPS.
https://arxiv.org/abs/1703.05175 - Carion, N., Massa, F., Synnaeve, G., Usunier, N., Kirillov, A., & Zagoruyko, S. (2020). End-to-End Object Detection with Transformers. ECCV.
https://arxiv.org/abs/2005.12872