ReNoise
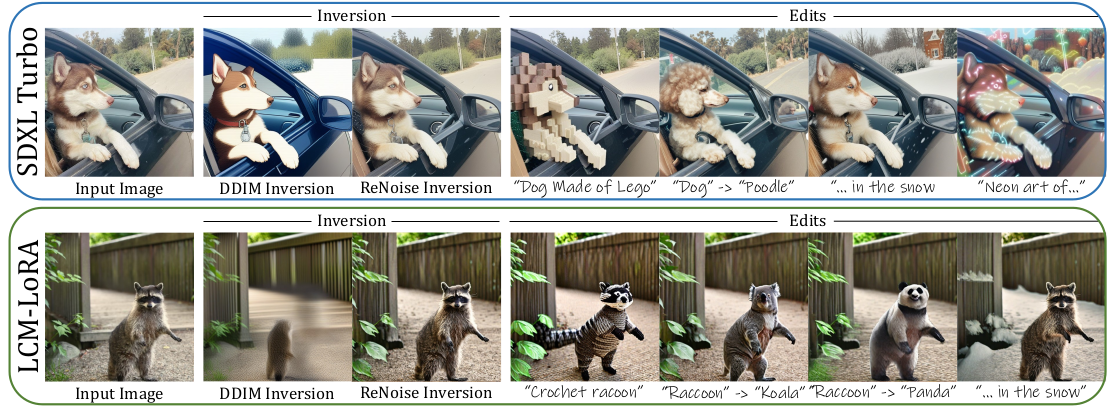
Revolutionizing Image Inversion and Editing with ReNoise
Recent advancements in text-guided diffusion models have unlocked powerful image manipulation capabilities. However, applying these methods to real images requires the challenging task of inverting images into the domain of pretrained diffusion models, particularly for newer models designed for a small number of denoising steps.
Introducing ReNoise
ReNoise inversion technique offers a high quality-to-operation ratio, significantly enhancing reconstruction accuracy without increasing the number of operations. By reversing the diffusion sampling process, this method employs an iterative renoising mechanism at each inversion sampling step. This approach refines the approximation of a predicted point along the forward diffusion trajectory by iteratively applying the pretrained diffusion model and averaging these predictions.
How It Works
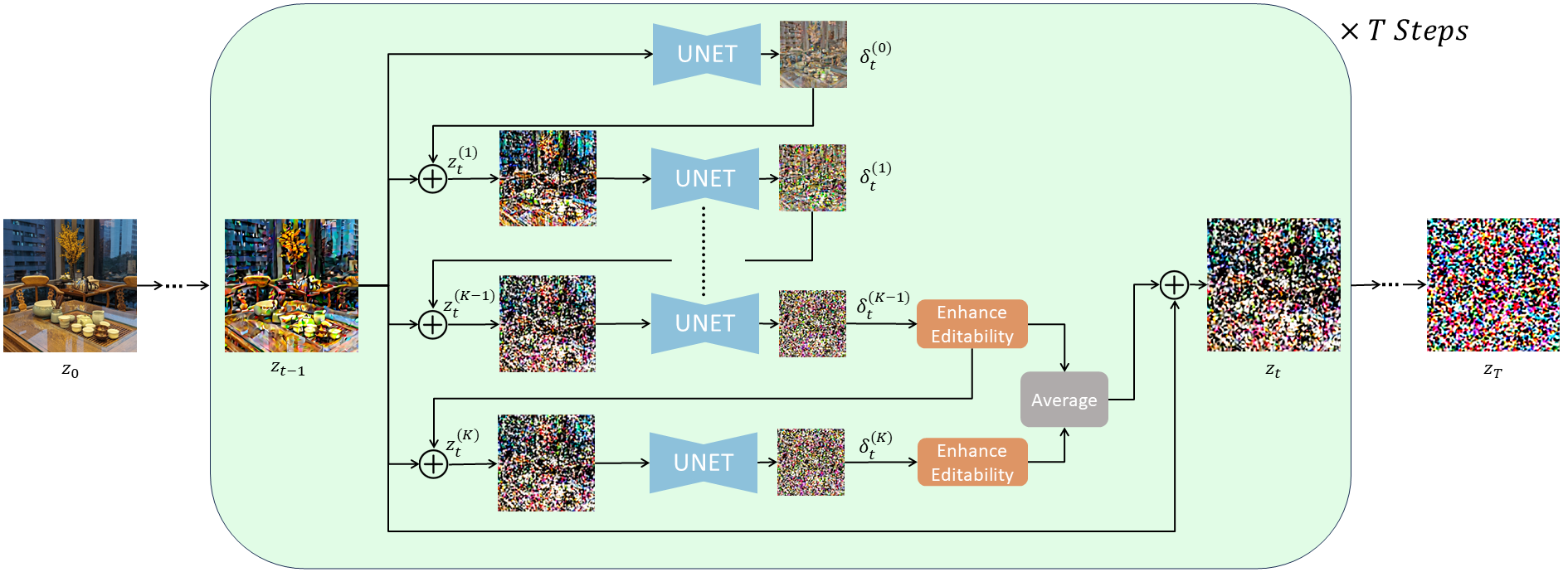
- Initial Approximation: Given an input image z0, we iteratively compute z1,z2,...,zT, where each zt is calculated from zt−1.
- Iterative Renoising: At each time step, we apply the UNet (ϵθ) K+1 times. The initial approximation is zt−1. The subsequent approximation zt(1) results from the reversed sampler step (e.g., DDIM). This process is repeated, progressively refining the approximation with each iteration.
- Optimization: For the last iterations, we optimize ϵθ(zt(k),t) to enhance editability. The final denoising direction is the average of the UNet predictions from the last few iterations.
- Final Output: This iterative process is repeated across multiple timesteps, resulting in the final inverted image zT.
Editing Results
Our approach not only improves reconstruction accuracy but also preserves editability. The quality of our inversions allows for prompt-driven image edits, enabling sophisticated and seamless text-driven manipulations on real images.
Performance Evaluation
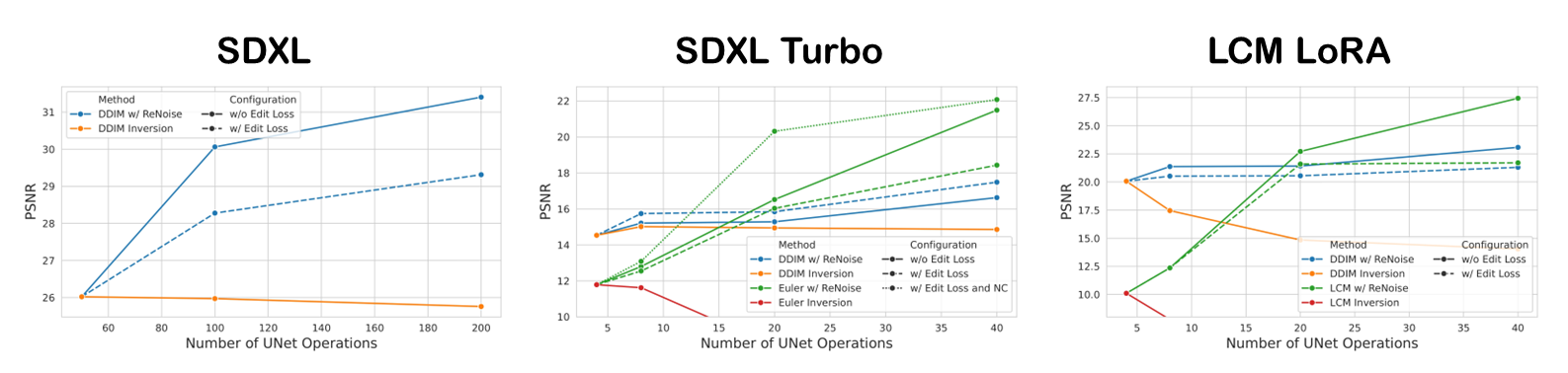
ReNoise performance were evaluated with various sampling algorithms and models, including recent accelerated diffusion models like SDXL Turbo and LCM. Comprehensive evaluations and comparisons demonstrate ReNoise's effectiveness in both accuracy and speed. Notably, this method outperforms traditional DDIM inversion, particularly in few-step models.
Conclusion
ReNoise represents a significant advancement in the field of image inversion and editing. By enhancing reconstruction accuracy and preserving editability, our technique paves the way for more effective and efficient text-guided diffusion models. Whether you're working with SDXL Turbo or LCM models, ReNoise offers a robust solution for high-quality image manipulation.