S-SYNTH : Knowledge-based synthetic generation of skin images
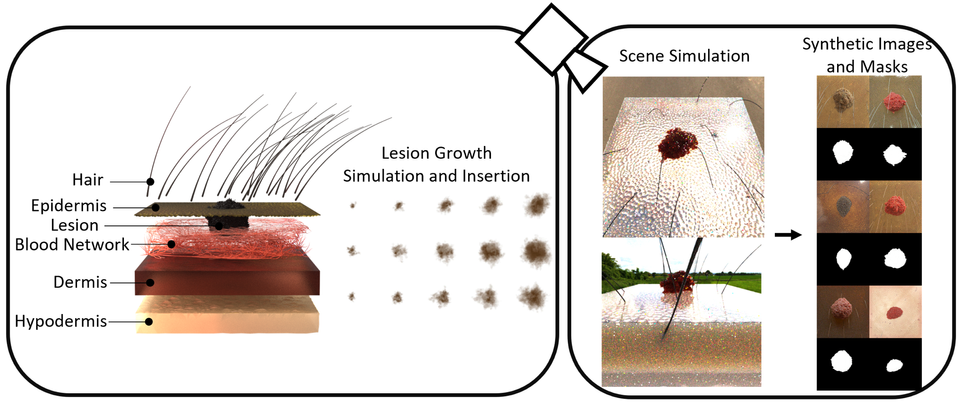
In the rapidly advancing field of dermatologic AI, access to large, diverse, and high-quality datasets is crucial for training models that are both robust and unbiased. However, this need is met with significant challenges, including the scarcity of annotated data and biases in existing datasets.
S-SYNTH is as an interesting and innovative framework that uses a knowledge-based approach to generate synthetic skin images. By creating anatomically accurate, diverse digital skin models, it proposes a new solution to the data problem in dermatologic AI.
The data problem in dermatologic AI
Building a robust, generalizable skin AI requires large datasets that represent the target populations and their subgroups. Unfortunately, current resources fall short in several critical ways :
Lack of data
- Public dermatologic datasets typically include few examples
- Only a small fraction of existing datasets include detailed annotations (e.g. pixel-level annotations for tasks like lesion segmentation).
Difficulty of annotation process by dermatologists
- Detailed annotation, often requiring dermatologists’ expertise, is a time-consuming and error-prone process.
- The lack of standardized annotation methods introduces variations among truthers and affects model reliability.
- There is also a lack of transparency concerning some crucial data information. Annotating skin tone, a vital parameter for model generalization, is often overlooked, with over 90% of studies failing to report this information (Daneshjou et al., 2021).
Bias in skin tone representation
- Lighter skin tones dominate publicly available datasets (Kinyanjui et al., 2019)
- This imbalance negatively impacts AI performance for darker skin tones, particularly in tasks such as lesion segmentation, where the models struggle with features unique to these populations (Benčević et al., 2024).
- More generally, public datasets do not equitably represent patient populations (Chen et al., 2023).
These challenges make it clear that alternative methods, such as synthetic data generation, are necessary to augment real-world datasets and improve AI models.
Related work in synthetic skin data generation
- Some GAN approach :
- Skin lesion synthesis [...] DCGAN classifier, Behara et al., 2023
- Controllable skin lesion synthesis [...] CGANs, Oliveira, 2020
- Some diffusion approaches :
- Augmenting medical image classifiers with synthetic data from latent diffusion models, Sagers et al., 2023
- Improving dermatology classifiers across populations using images generated by large diffusion models, Sagers et al, 2022
- Style transfer & deep blending to improve lesion classification accuracy by increasing skin color diversity (Rezk et al., 2022)
Synthetic data offers promising solutions, unfortunately it comes with its own set of limitations such as :
- Technical complexity : models like GANs require tuning of hyperparameters and supervision.
- Artifacts in training sets : these methods often carry over flaws from real-world datasets into synthetic ones.
- Generalization challenges : simulated images may fail to capture the full range of physical and morphological variations in diseases and acquisition systems.
Unlike GANs or diffusion models, S-SYNTH uses a knowledge-based approach, addressing the shortcomings of previous methods.
S-SYNTH overview
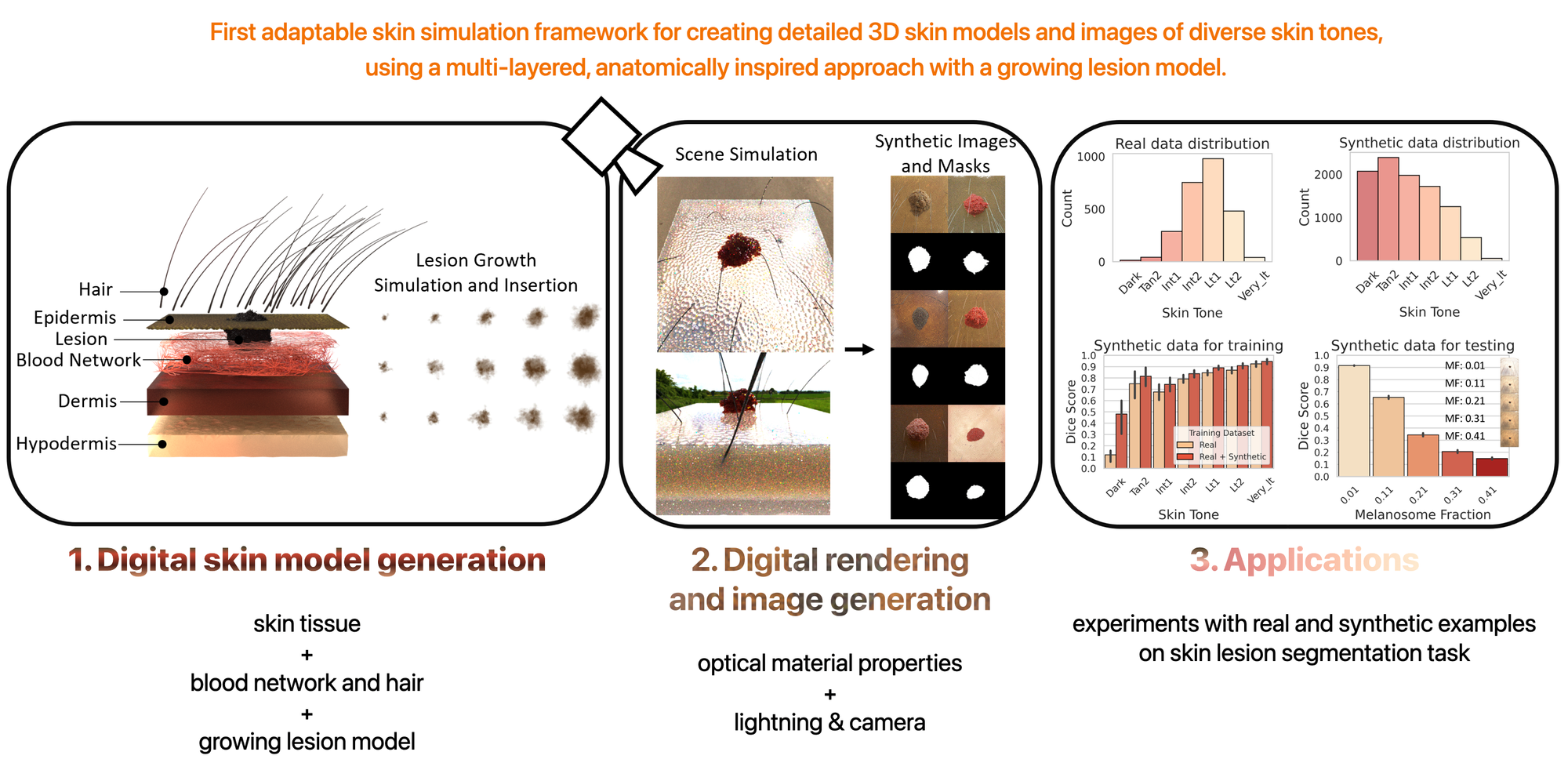
- Digital Skin Modeling :
- S-SYNTH generates multi-layered skin models, representing the epidermis, dermis, and hypodermis with realistic thicknesses and textures.
- Hair properties, including density, length, and curvature, are accurately modeled, adding further realism.
- Lesion Growth Simulation :
Lesions are dynamically grown within the skin model using biologically inspired algorithms. These account for irregular growth patterns, mimicking real-world conditions such as uneven lesion boundaries.
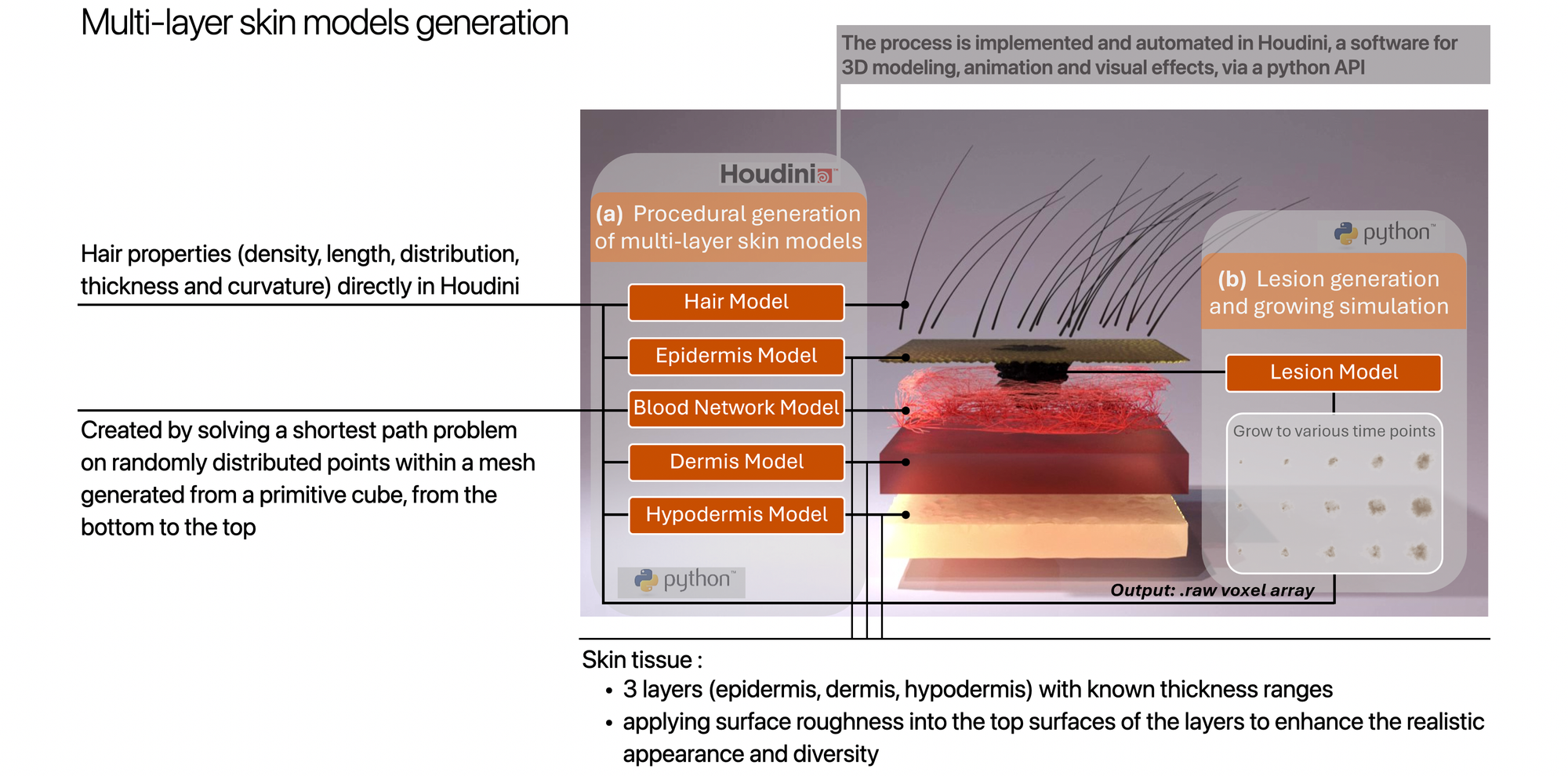
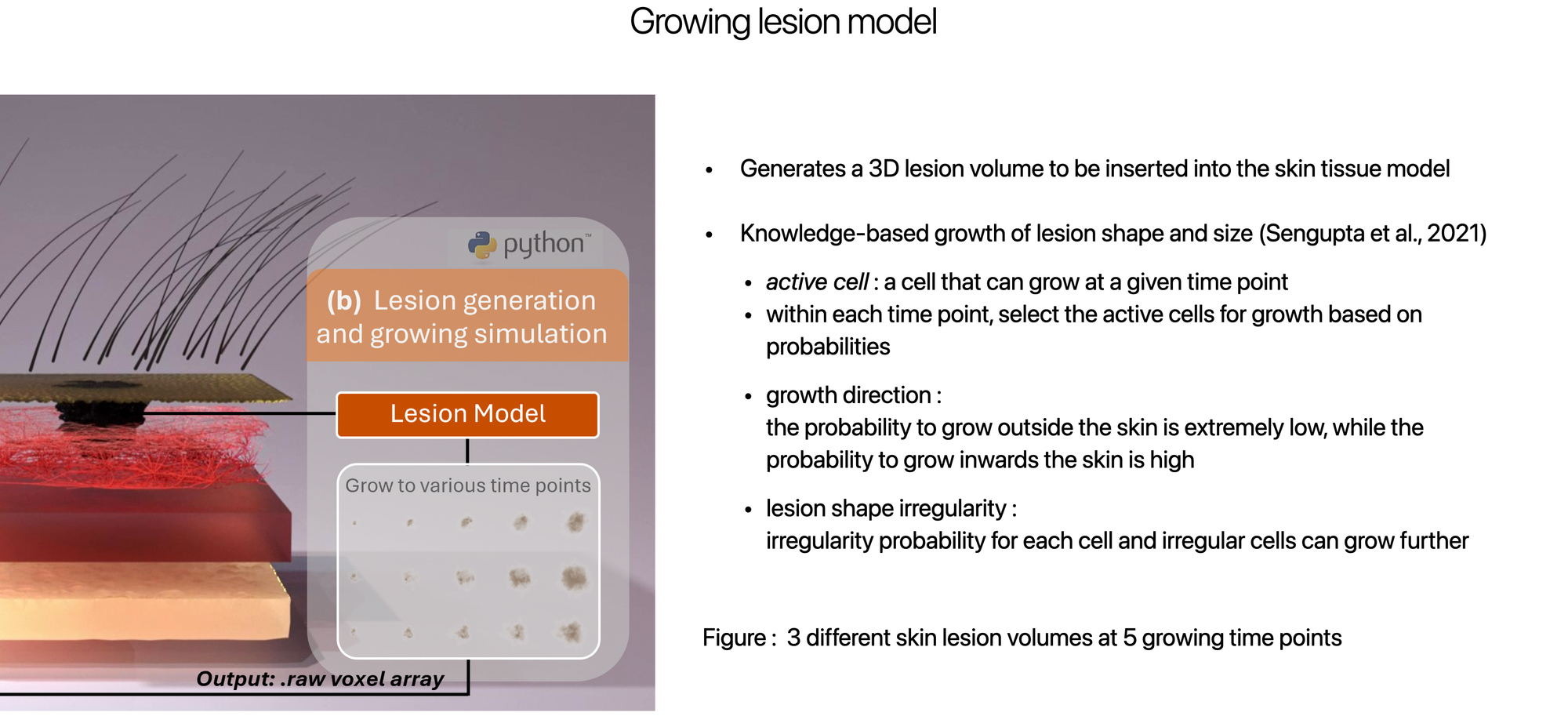
Please click to enlarge the images.
3. Digital rendering and image generation :
- S-SYNTH assigns optical properties like absorption and scattering coefficients to different skin layers, ensuring accurate light-skin interactions.
- A volumetric path tracer simulates lighting conditions and camera settings, producing high-resolution realistic synthetic images.
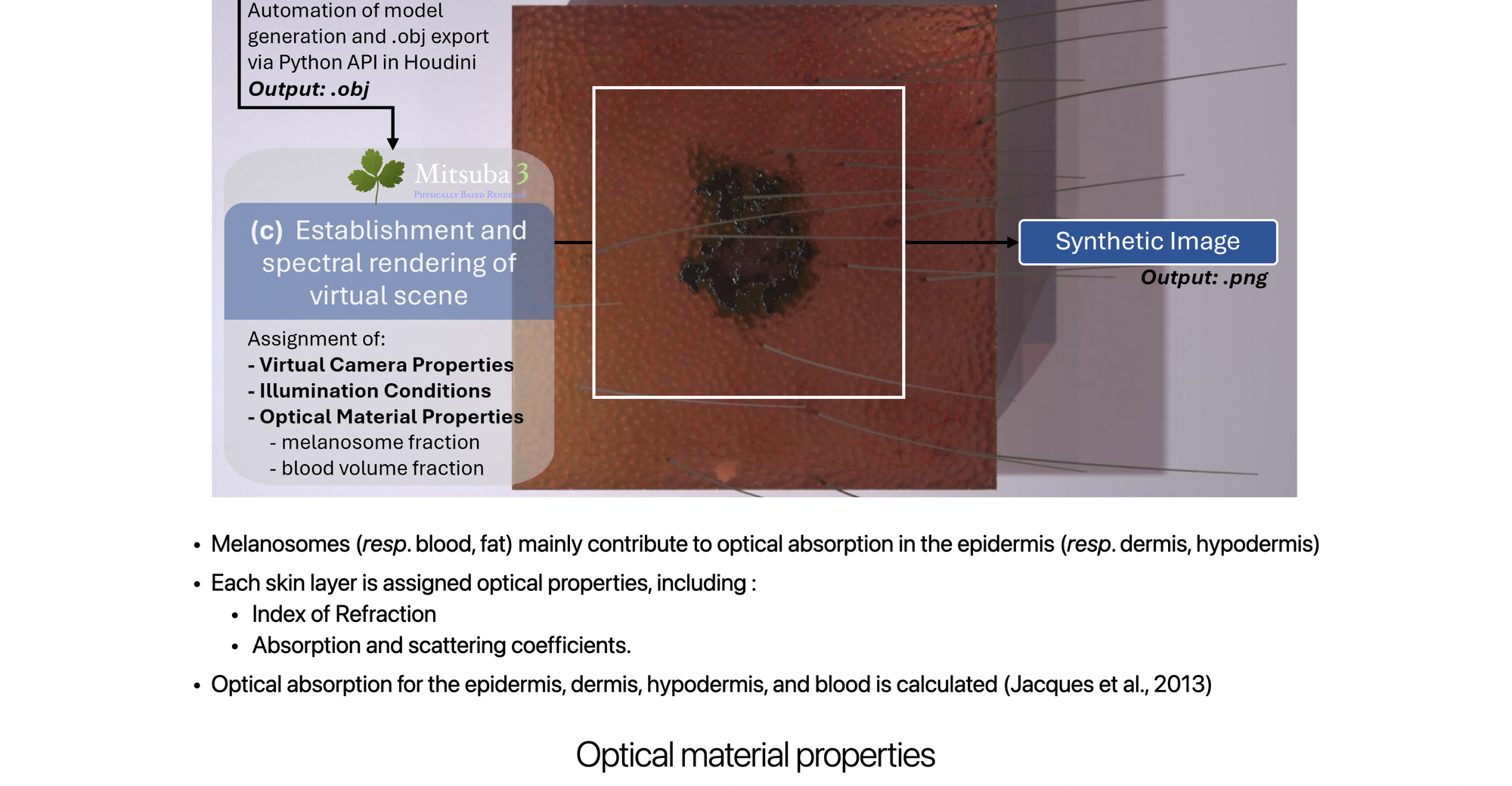
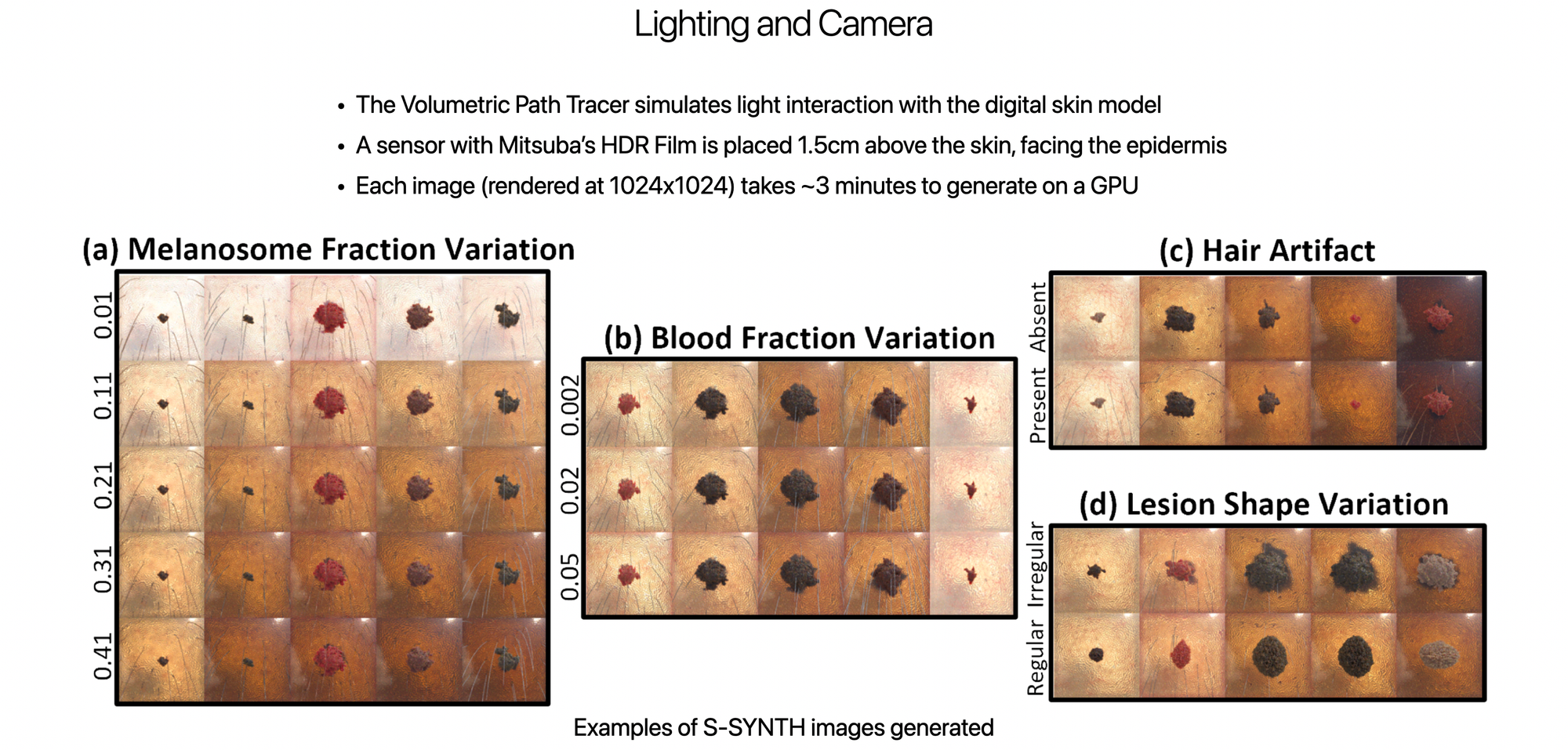
Please click to enlarge the images.
S-SYNTH overcome the AI-based methods limitations by using a knowledge-based approach, ensuring images are anatomically grounded and optically precise.
Applications and experiments
S-SYNTH has been extensively tested in experiments combining real and synthetic datasets for skin lesion segmentation tasks, using DermoSegDiff, a SoTA diffusion-based skin segmentation method, differentiates lesions from background.
Here's some information about the datasets that were used for the experiments :
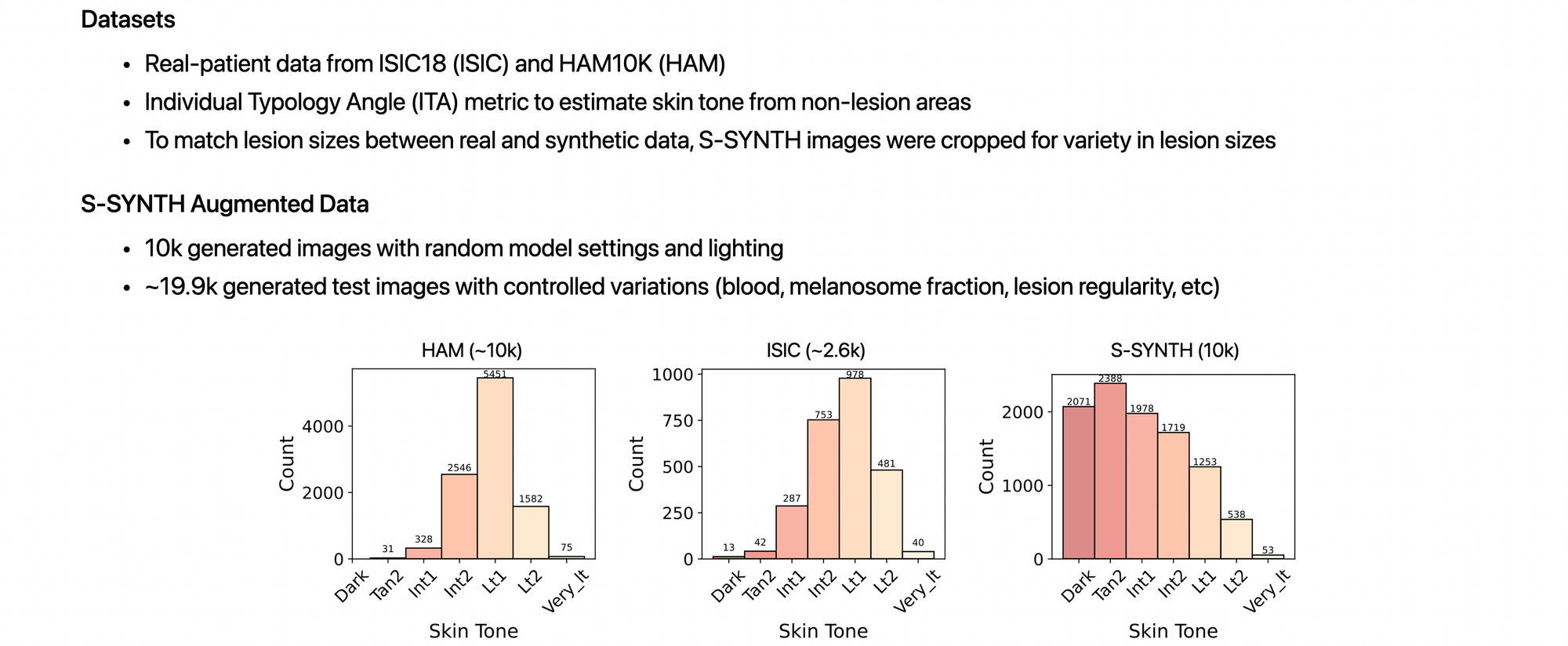
The findings are promising:
Enhancing skin lesion segmentation
1. Augmenting Real Data
- When S-SYNTH-generated images were added to training sets, the models showed improved accuracy, particularly for darker skin tones.
- Models trained on a mix of real and synthetic data outperformed those trained on real data alone.
2. Testing Biases
Models performed differently based on specific synthetic image properties :
- Melanosome Fraction : higher concentrations (indicative of darker skin tones) led to a drop in performance, reflecting biases in dermatologic AI.
- Lesion Shape : irregularly shaped lesions posed greater challenges for models compared to regular ones.
3. Limitations of Exclusively Synthetic Training:
Training models solely on synthetic data reduced performance due to residual differences between real and synthetic images. This underscores the need for balanced datasets that include both real and synthetic examples.
Bridging the gap for darker skin tones
One of the most impactful outcomes of S-SYNTH is its ability to address biases in AI performance for darker skin tones. By generating diverse skin tones and lesion types, S-SYNTH helps create datasets that reflect real-world diversity, ensuring more equitable AI outcomes.
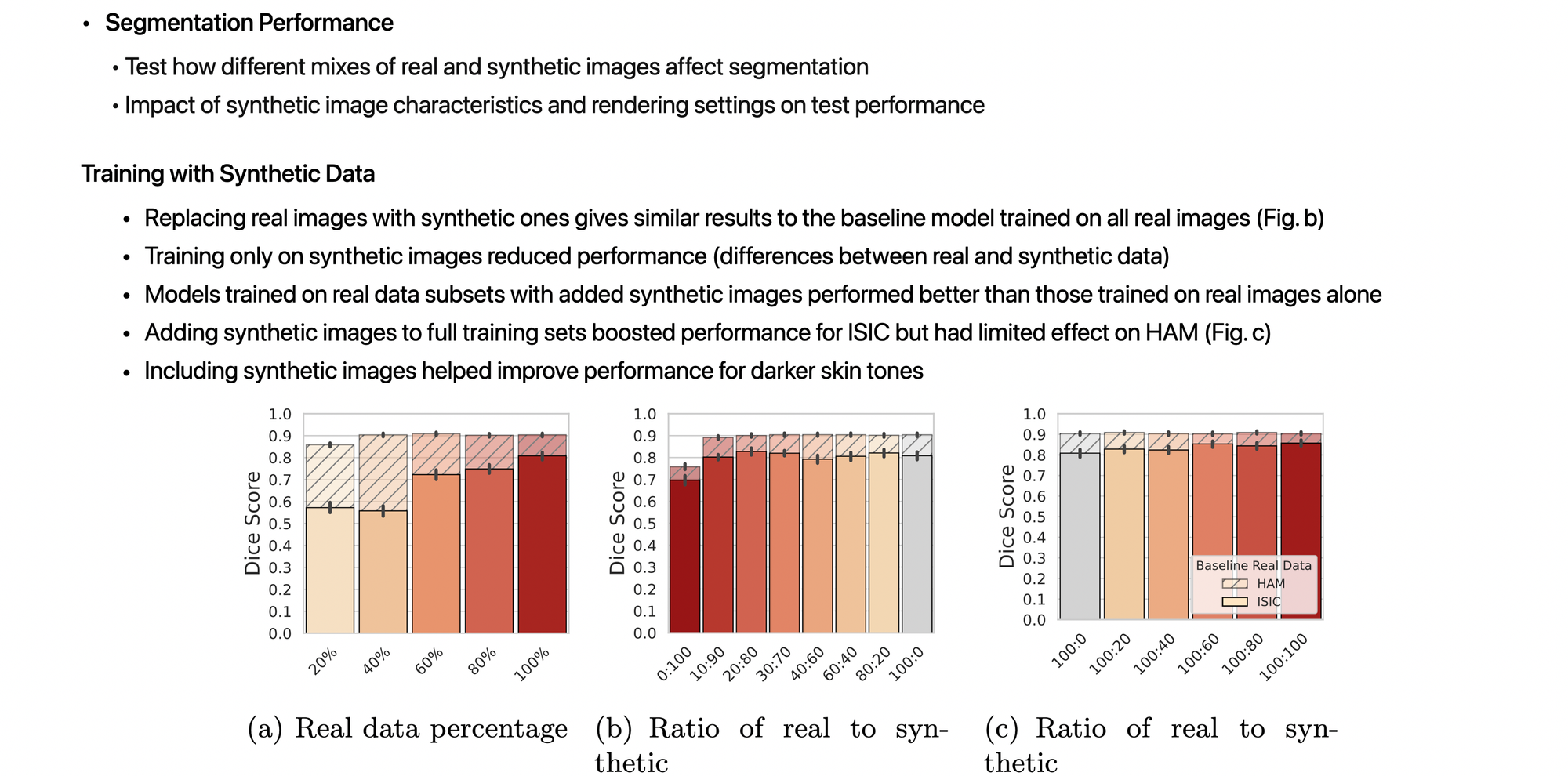
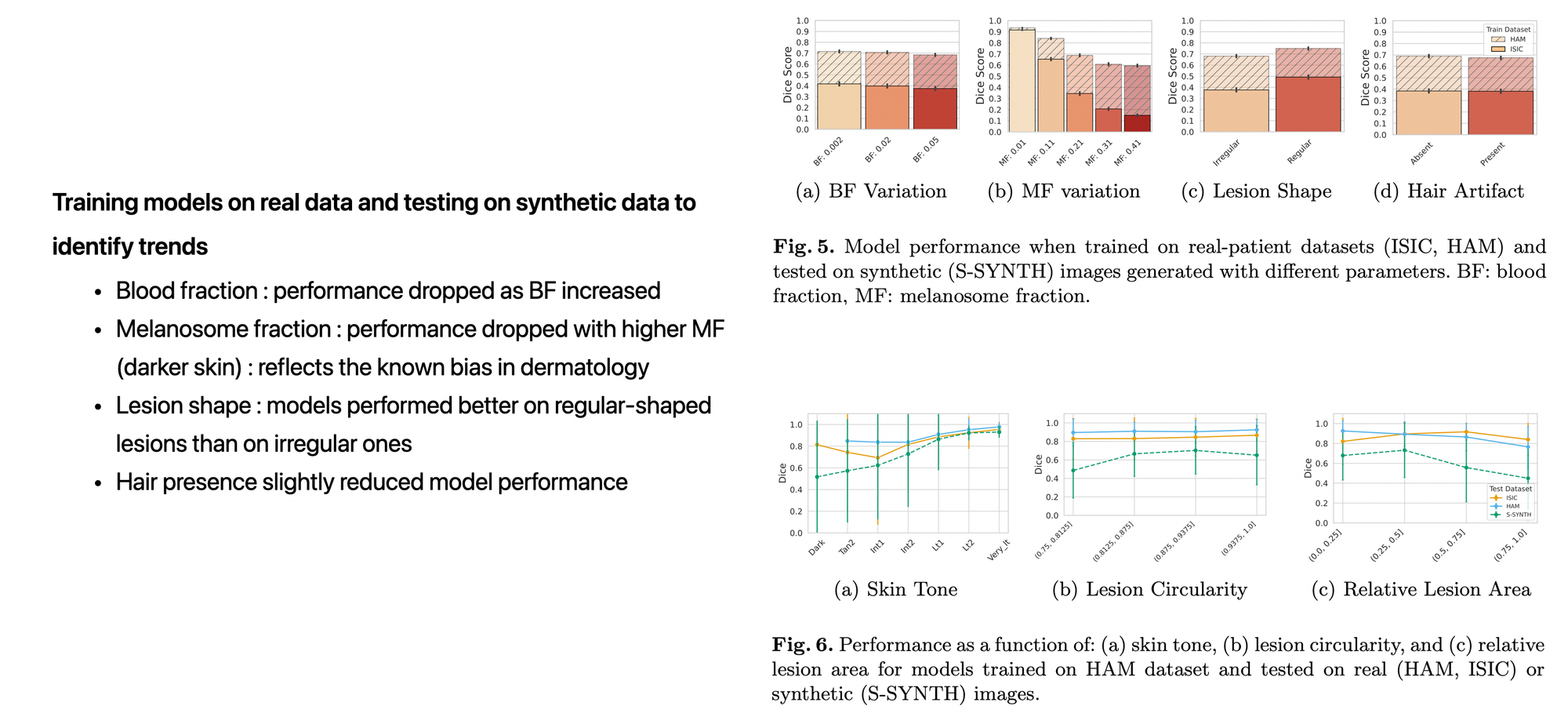
Please click to enlarge the images.
Conclusion
S-SYNTH sets a new standard for synthetic data generation in medical AI.
By generating realistic, diverse synthetic skin images, it addresses critical gaps in dataset availability and bias, empowering researchers to develop AI models that are not only more accurate but also more inclusive.
As S-SYNTH continues to evolve, it could play a pivotal role in democratizing access to high-quality medical AI tools, ensuring that these technologies benefit diverse populations equitably.