Diffuse, Attend, and Segment: Unsupervised Zero-Shot Segmentation using Stable Diffusion
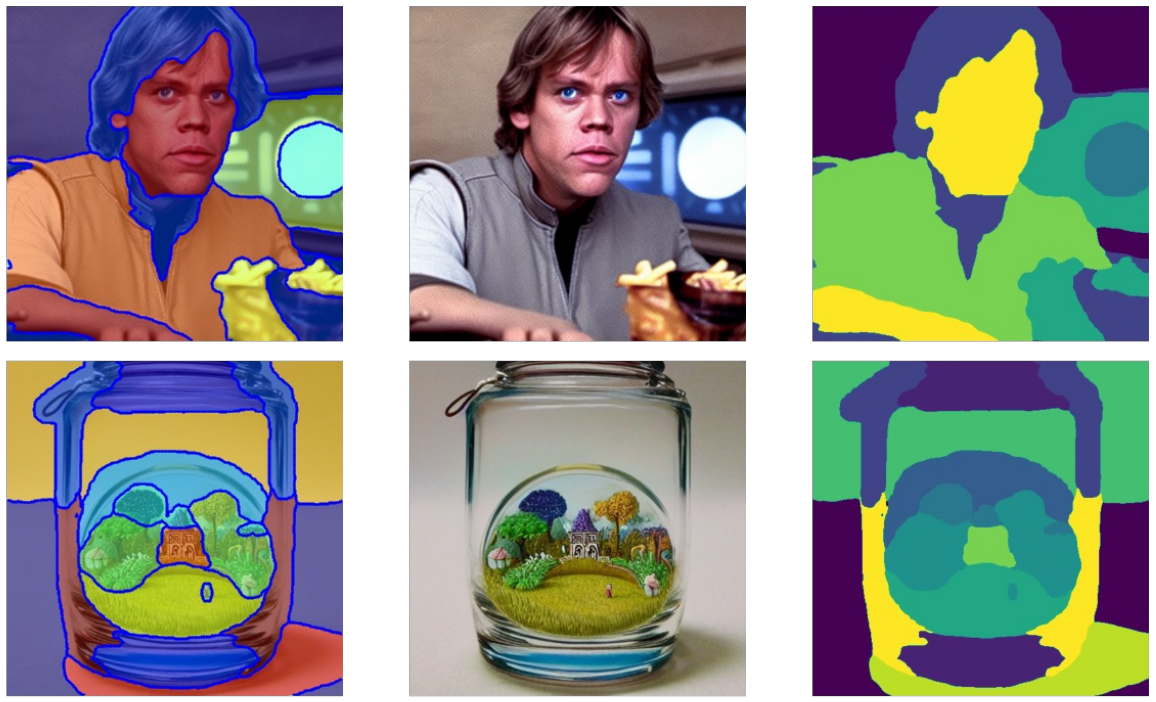
Introduction
In computer vision, creating accurate segmentation masks for images remains a challenging task. Recent advancements in supervised and unsupervised training methods have made strides, yet achieving zero-shot segmentation without annotations poses a considerable challenge. A promising breakthrough has emerged, harnessing stable diffusion models and their self-attention layers. These pre-trained models learn object concepts within their attention layers, offering a path to zero-shot segmentation.
Methodology
Enter DiffSeg, a novel method leveraging stable diffusion models for unsupervised zero-shot segmentation. By tapping into the inherent concepts learned by pre-trained models, DiffSeg uses self-attention layers with Intra-Attention Similarity and Inter-Attention Similarity to uncover segmentation masks. It employs a three-step process involving attention aggregation, iterative attention merging, and non-maximum suppression.
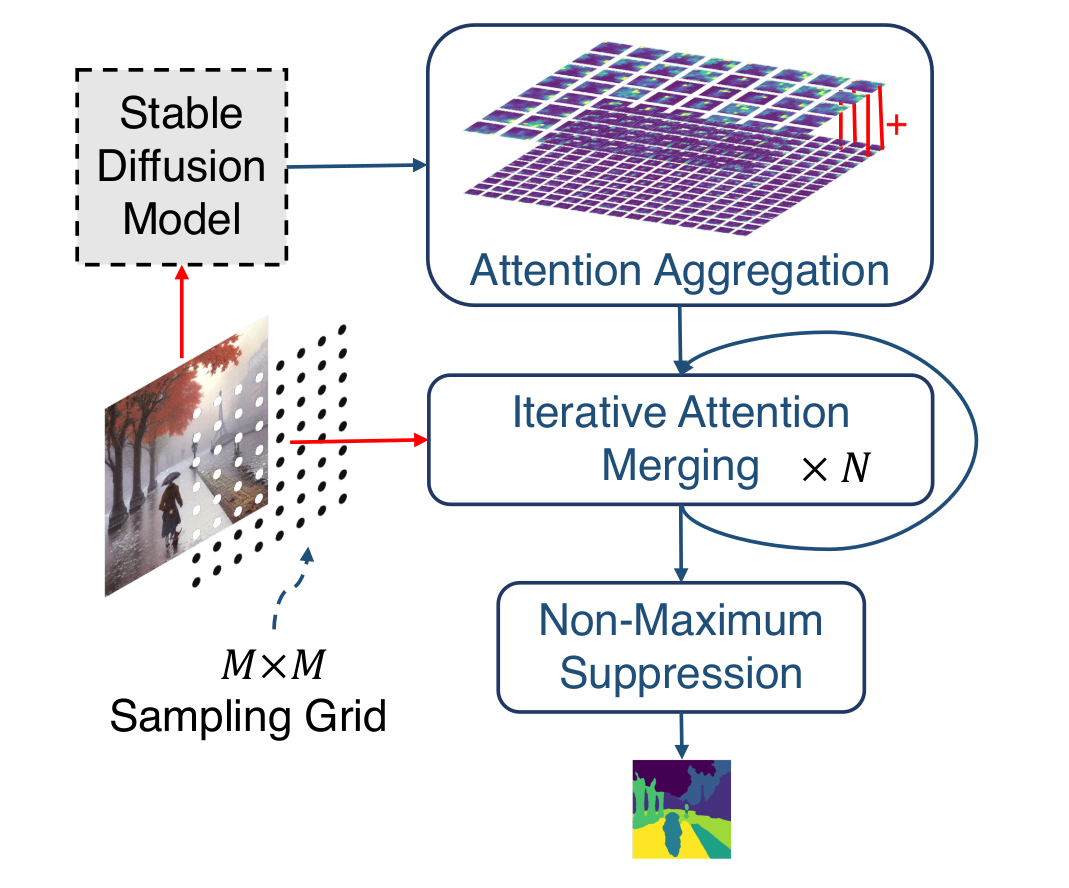
Results and Evaluation
DiffSeg showcases state-of-the-art performance on benchmark datasets like COCO-Stuff-27 and Cityscapes. Outperforming prior methods by a significant margin, it achieves a 26% improvement in pixel accuracy and a 17% increase in mean intersection over union (mIoU) on COCO-Stuff-27. On Cityscapes, DiffSeg matches or outperforms existing methods, highlighting its robustness across diverse datasets.
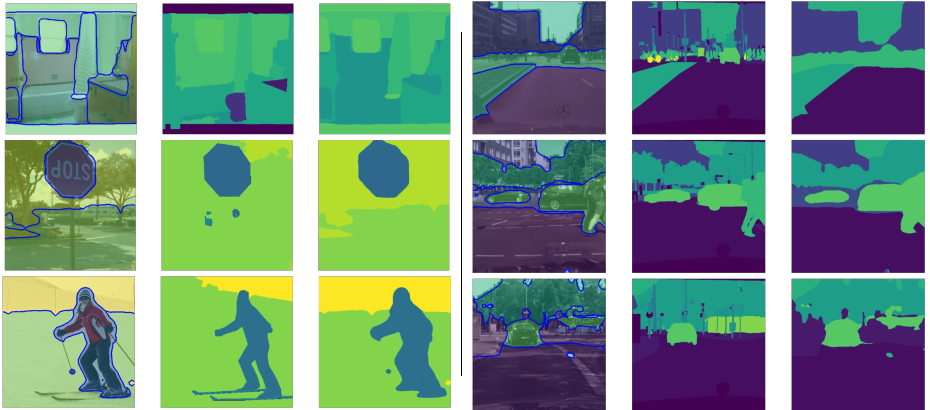
Conclusion
DiffSeg is a novel unsupervised zero-shot image segmentation method driven by a pre-trained stable diffusion model. Requiring no prior knowledge or external resources, it stands out for its state-of-the-art performance on popular benchmarks and superior generalization across diverse image styles. With competitive results on COCO-Stuff-27 and Cityscapes, DiffSeg is deterministic and does not demand specifying the number of clusters in advance. While not real-time and influenced by model size, DiffSeg emerges as a promising approach for unsupervised and zero-shot image segmentation.
Reference
Tian, J., Aggarwal, L., Colaco, A., Kira, Z., & Gonzalez-Franco, M. (2023). Diffuse, Attend, and Segment: Unsupervised Zero-Shot Segmentation using Stable Diffusion.