"MAGVIT: Masked Generative Video Transformer"
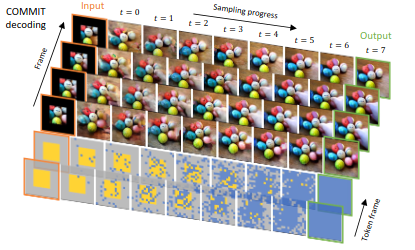
#ComputerVision #GenerativeVideo #Transformers #MaskedGit
Introduction
With the advances of technologies for images and video generation, researchers offered a new model of video generation based on vision transformers.
MAGVIT became the new state-of-the-art in the domain of generative video with its release on 10th December 2022.
It is faster than other models and can do multiple tasks like Frame Prediction or Frame Interpolation with one single model.
The Transformers are some models developped originally to solve sequence transduction. They were really good when it comes to speech recognition or text-to-speech transformation. After showing their performances in those domains, researchers tried to use it on images. We call it Vision Transformers.
MAGVIT overview
The MAGVIT model is a multitask model which can perform Frame Prediction, Frame Interpolation, Outpainting, Inpainting, Class-conditionnal Generation and some other tasks.
It takes one or multiple images (based on task) as input and generates video.
We can define different vocabulary:
- Encoder : Neural network that transforms any type of sequence to another sequence of numbers. For example, it will transform this phrase or an image into numbers.
- Decoder : Neural network that transforms a sequence of number into any type of sequence.
Architecture
The MAGVIT is based on 2 parts with autoregressive transformers:
- A Vector-Quantized (VQ) auto-encoder
- A masked token modeling (MTM)
The VQ is used to encode the images to vectors and the MTM is used to predict videos based on encoded images.
Image from https://openaccess.thecvf.com/content/CVPR2023/papers/Yu_MAGVIT_Masked_Generative_Video_Transformer_CVPR_2023_paper.pdf
The COMMIT Method
The MTM was good but not flexible and there was a lack of generalization. Then, they implemented a new method : The Conditional Masked Modeling by Interior Tokens (COMMIT). It first embeds the image by the VQ then it changes the token into conditional token, masking it or keeping it based on conditional function.
This lets the model generalize more.
Image from https://openaccess.thecvf.com/content/CVPR2023/papers/Yu_MAGVIT_Masked_Generative_Video_Transformer_CVPR_2023_paper.pdf
Results and Conlusion
Image from https://openaccess.thecvf.com/content/CVPR2023/papers/Yu_MAGVIT_Masked_Generative_Video_Transformer_CVPR_2023_paper.pdf
As we can see above, MAGVIT is performing well on frame prediction. It is the best for multiples tasks. It is at the state of art for all the tasks we speak about.
In conclusion, the MAGVIT is:
- at the state of art for multiples tasks (10 or less)
- able to generalize for each task
- faster than others models
- using a COMMIT method which dramatically augments its generalization
References
https://openaccess.thecvf.com/content/CVPR2023/papers/Yu_MAGVIT_Masked_Generative_Video_Transformer_CVPR_2023_paper.pdf