Physics-Constrained Gaussian Process Regression
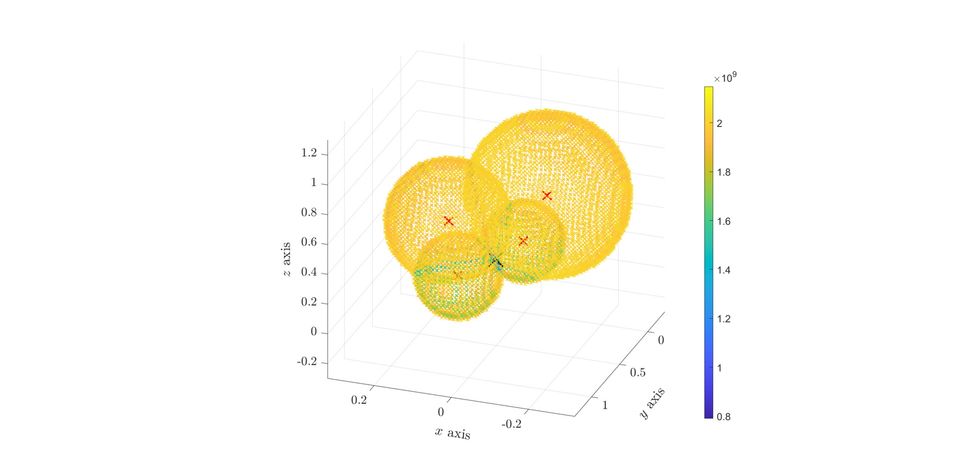
Iain HENDERSON
PhD, INSA, Toulouse
April 20th, 2023
Abstract
Gaussian process regression (GPR) is a flexible regression method especially suited to scarce data regimes. It is a Bayesian and nonparametric regression method, which consists in conditioning the probability law of a Gaussian stochastic process on a given data set, and then in using the law of the conditioned process to regress the target function. It is alternatively understood as a kernel method. In parallel, physical models typically take the form of partial differential equations (PDEs). The modern theory of PDEs is built on functional analysis, which has proved its efficiency both for the theoretical study and the numerical solving of PDEs.
In this presentation, we will tackle the question of using GPR to solve a number of problems controlled by PDEs, by enforcing the PDE constraints directly on the Gaussian process, leading to a "physics-informed" GPR. In order to provide a sound framework for this approach, we will provide some theoretical results describing how to rigorously impose certain physical constraints (explicitly, the strict PDE constraint if the PDE is linear, and the control of the Sobolev energy norm) on the underlying Gaussian process.
We will then provide a simple application test case, with the estimation of the solution of the 3D wave equation (central in acoustics), as well as the estimation of the physical parameters attached to this PDE. We will finish with providing some outlooks concerning nonlinear PDEs in particular. Short discussions with (physics-informed) neural networks will also be provided.
This work is done as part of a PhD thesis advised by Pascal Noble (IMT/INSA) and Olivier Roustant (IMT/INSA), and funded by the Service Hydrographique et Océanographique de la Marine (SHOM).